Journal Description
Remote Sensing
Remote Sensing
is a peer-reviewed, open access journal about the science and application of remote sensing technology, and is published semimonthly online by MDPI. The Remote Sensing Society of Japan (RSSJ) and the Japan Society of Photogrammetry and Remote Sensing (JSPRS) are affiliated with Remote Sensing, and their members receive a discount on the article processing charge.
- Open Access— free for readers, with article processing charges (APC) paid by authors or their institutions.
- High Visibility: indexed within Scopus, SCIE (Web of Science), Ei Compendex, PubAg, GeoRef, Astrophysics Data System, Inspec, dblp, and other databases.
- Journal Rank: JCR - Q1 (Geosciences, Multidisciplinary) / CiteScore - Q1 (General Earth and Planetary Sciences)
- Rapid Publication: manuscripts are peer-reviewed and a first decision is provided to authors approximately 21.1 days after submission; acceptance to publication is undertaken in 2.6 days (median values for papers published in this journal in the first half of 2023).
- Recognition of Reviewers: reviewers who provide timely, thorough peer-review reports receive vouchers entitling them to a discount on the APC of their next publication in any MDPI journal, in appreciation of the work done.
- Companion journal: Geomatics
Impact Factor:
5.0 (2022);
5-Year Impact Factor:
5.6 (2022)
Latest Articles
Direct Assimilation of Ground-Based Microwave Radiometer Clear-Sky Radiance Data and Its Impact on the Forecast of Heavy Rainfall
Remote Sens. 2023, 15(17), 4314; https://doi.org/10.3390/rs15174314 (registering DOI) - 01 Sep 2023
Abstract
Ground-based microwave radiometer (GMWR) data with high spatial and temporal resolution can improve the accuracy of weather forecasts when effectively assimilated into numerical weather prediction. Nowadays, the major method to assimilate these data is via indirect assimilation by assimilating the retrieved profiles, which
[...] Read more.
Ground-based microwave radiometer (GMWR) data with high spatial and temporal resolution can improve the accuracy of weather forecasts when effectively assimilated into numerical weather prediction. Nowadays, the major method to assimilate these data is via indirect assimilation by assimilating the retrieved profiles, which introduces large retrieval errors and cannot easily be represented by an error covariance matrix. Direct assimilation, on the other hand, can avoid this issue. In this study, the ground-based version of the Radiative Transfer for the TIROS Operational Vertical Sounder (RTTOV-gb) was selected as the observation operator, and a direct assimilation module for GMWR radiance data was established in the Weather Research and Forecasting Model Data Assimilation (WRFDA). Then, this direct assimilation module was applied to assimilate GMWR data. The results were compared to the indirect assimilation experiment and demonstrated that direct assimilation can more effectively improve the model’s initial fields in terms of temperature and humidity than indirect assimilation while avoiding the influence of retrieval errors. In addition, direct assimilation performed better in the precipitation forecast than indirect assimilation, making the main precipitation center closer to the observation. In particular, the improvement in the precipitation forecast with a threshold of 60 mm/6 h was obvious, and the corresponding TS score was significantly enhanced.
Full article
(This article belongs to the Special Issue Remote Sensing Data Application, Data Reanalysis and Advances for Mesoscale Numerical Weather Models)
►
Show Figures
Open AccessArticle
The Impact Mechanism of Climate and Vegetation Changes on the Blue and Green Water Flow in the Main Ecosystems of the Hanjiang River Basin, China
Remote Sens. 2023, 15(17), 4313; https://doi.org/10.3390/rs15174313 (registering DOI) - 01 Sep 2023
Abstract
Water resources management and planning traditionally focus on visible liquid or blue water. However, green water also maintains social development and ecosystem services. Therefore, blue and green water should be incorporated into the watershed management system for evaluating water resources. To analyze the
[...] Read more.
Water resources management and planning traditionally focus on visible liquid or blue water. However, green water also maintains social development and ecosystem services. Therefore, blue and green water should be incorporated into the watershed management system for evaluating water resources. To analyze the water resources of the Hanjiang River Basin, the SWAT model was set up using long-term and high-precision geographic data. The methods of wavelet analysis and Pearson’s correlation analysis were used to explore the influence mechanism of climate and vegetation changes on the blue and green water flow (BWF and GWF) of the main ecosystems in the basin. The results showed that: (1) The spatial–temporal distribution of the BWF and GWF in the main ecosystems of the basin over the past 50 years was uneven. Forest ecosystems and farmland ecosystems have a greater concentration of water resources in the south, while grassland ecosystems have a greater concentration of water resources in the east. (2) Climate dominates the BWF and GWF changes in the main ecosystems of the basin. The BWF and the precipitation change cycle are synergistic, and the GWF and the temperature change cycle are synergistic. (3) The correlation between vegetation and BWF and GWF in the farmland ecosystem is significant. Vegetation affects the hydrological change process of the BWF and GWF at the microscale. This study can provide data support and scientific rules for ecosystem water resource management in the basin.
Full article
(This article belongs to the Special Issue Remote Sensing in Natural Resource and Water Environment II)
►▼
Show Figures
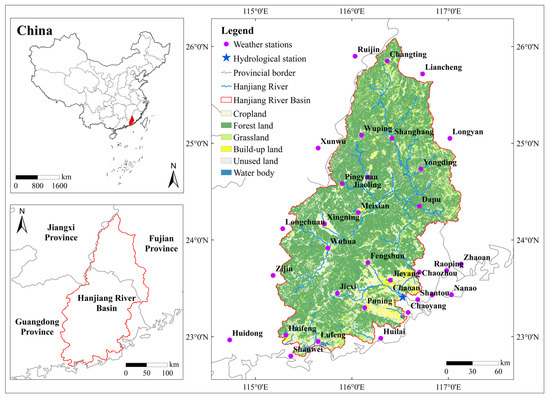
Figure 1
Open AccessArticle
A UAV Path Planning Method for Building Surface Information Acquisition Utilizing Opposition-Based Learning Artificial Bee Colony Algorithm
Remote Sens. 2023, 15(17), 4312; https://doi.org/10.3390/rs15174312 (registering DOI) - 01 Sep 2023
Abstract
To obtain more building surface information with fewer images, an unmanned aerial vehicle (UAV) path planning method utilizing an opposition-based learning artificial bee colony (OABC) algorithm is proposed. To evaluate the obtained information, a target information entropy ratio model based on observation angles
[...] Read more.
To obtain more building surface information with fewer images, an unmanned aerial vehicle (UAV) path planning method utilizing an opposition-based learning artificial bee colony (OABC) algorithm is proposed. To evaluate the obtained information, a target information entropy ratio model based on observation angles is proposed, considering the observation angle constraints under two conditions: whether there is an obstacle around the target or not. To efficiently find the optimal observation angles, half of the population that is lower-quality generates bit points through opposition-based learning. The algorithm searches for better individuals near the bit points when generating new solutions. Furthermore, to prevent individuals from observing targets repeatedly from similar angles, the concept of individual abandonment probability is proposed. The algorithm can adaptively abandon similar solutions based on the relative position between the individual and the population. To verify the effectiveness of the proposed method, information acquisition experiments were conducted on real residential buildings, and the results of 3D reconstruction were analyzed. The experiment results show that while model accuracy is comparable to that of the comparison method, the number of images obtained is reduced to one-fourth of the comparison method. The operation time is significantly reduced, and 3D reconstruction efficiency is remarkably improved.
Full article
(This article belongs to the Special Issue Trends, Innovative Developments and Disruptive Applications in UAV Remote Sensing)
►▼
Show Figures
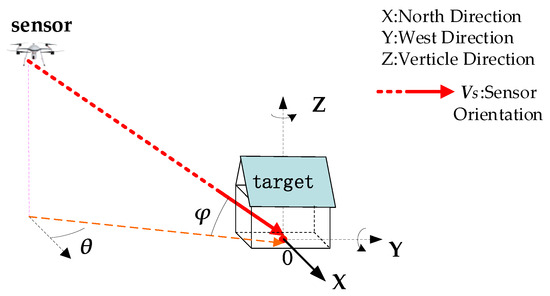
Figure 1
Open AccessArticle
Hierarchical Edge-Preserving Dense Matching by Exploiting Reliably Matched Line Segments
Remote Sens. 2023, 15(17), 4311; https://doi.org/10.3390/rs15174311 (registering DOI) - 01 Sep 2023
Abstract
Image dense matching plays a crucial role in the reconstruction of three-dimensional models of buildings. However, large variations in target heights and serious occlusion lead to obvious mismatches in areas with discontinuous depths, such as building edges. To solve this problem, the present
[...] Read more.
Image dense matching plays a crucial role in the reconstruction of three-dimensional models of buildings. However, large variations in target heights and serious occlusion lead to obvious mismatches in areas with discontinuous depths, such as building edges. To solve this problem, the present study mines the geometric and semantic information of line segments to produce a constraint for the dense matching process. First, a disparity consistency-based line segment matching method is proposed. This method correctly matches line segments on building structures in discontinuous areas based on the assumption that, within the corresponding local areas formed by two corresponding line pairs, the disparity obtained by the coarse-level matching of the hierarchical dense matching is similar to that derived from the local homography estimated from the corresponding line pairs. Second, an adaptive guide parameter is designed to constrain the cost propagation between pixels in the neighborhood of line segments. This improves the rationality of cost aggregation paths in discontinuous areas, thereby enhancing the matching accuracy near building edges. Experimental results using satellite and aerial images show that the proposed method efficiently obtains reliable line segment matches at building edges with a matching precision exceeding 97%. Under the constraint of the matched line segments, the proposed dense matching method generates building edges that are visually clearer, and achieves higher accuracy around edges, than without the line segment constraint.
Full article
(This article belongs to the Special Issue 3D Information Recovery and 2D Image Processing for Remotely Sensed Optical Images)
►▼
Show Figures
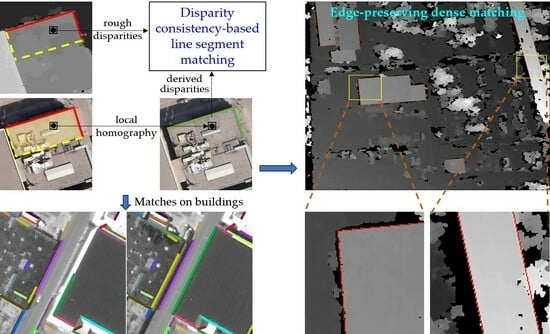
Graphical abstract
Open AccessTechnical Note
Select Informative Samples for Night-Time Vehicle Detection Benchmark in Urban Scenes
Remote Sens. 2023, 15(17), 4310; https://doi.org/10.3390/rs15174310 (registering DOI) - 31 Aug 2023
Abstract
Night-time vehicle detection plays a vital role due to the high incidence of abnormal events in our daily security field. However, existing studies mainly focus on vehicle detection in autonomous driving and traffic intersection scenes, but ignore urban scenes. There are vast differences
[...] Read more.
Night-time vehicle detection plays a vital role due to the high incidence of abnormal events in our daily security field. However, existing studies mainly focus on vehicle detection in autonomous driving and traffic intersection scenes, but ignore urban scenes. There are vast differences between these scenes, such as viewpoint, position, illumination, etc. In this paper, the authors present a night-time vehicle detection dataset collected from urban scenes, named Vehicle Detection in Night-Time Urban Scene (VD-NUS). The VD-NUS dataset consists of more than 100 K challenging images, comprising a total of about 500 K labelled vehicles. This paper introduces a vehicle detection framework via an active auxiliary mechanism (AAM) to reduce the annotation workload. The proposed AAM framework can actively select the informative sample for annotation by estimating its uncertainty and locational instability. Furthermore, this paper proposes a computer-assisted detection module embedded in the AAM framework to help human annotators to rapidly and accurately label the selected data. AAM outperformed the baseline method (random sampling) by up to 0.91 AP and 3.0 MR−2 on the VD-NUS dataset.
Full article
(This article belongs to the Section Remote Sensing Image Processing)
►▼
Show Figures
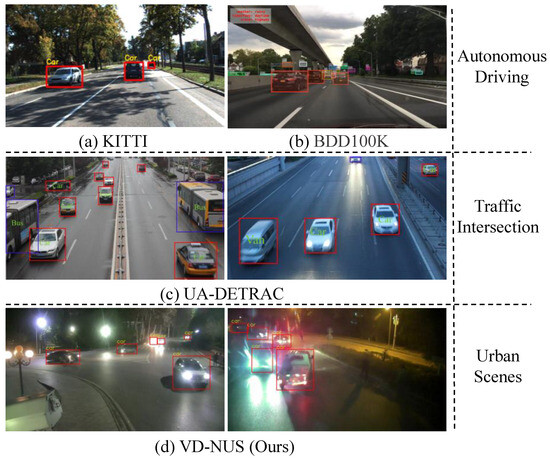
Figure 1
Open AccessArticle
A Quasi-Coherent Detection Method Based on Radon–Fourier Transform Using Multi-Frequency-Based Passive Bistatic Radar
Remote Sens. 2023, 15(17), 4309; https://doi.org/10.3390/rs15174309 (registering DOI) - 31 Aug 2023
Abstract
Passive bistatic radar (PBR)-based moving target detection (MTD) has benefited greatly from multi-frequency (MF) integration, which can effectively improve the detection capability of weak targets. However, with the increase in the coherent processing interval (CPI) and carrier-frequency separation, Doppler spread will appear in
[...] Read more.
Passive bistatic radar (PBR)-based moving target detection (MTD) has benefited greatly from multi-frequency (MF) integration, which can effectively improve the detection capability of weak targets. However, with the increase in the coherent processing interval (CPI) and carrier-frequency separation, Doppler spread will appear in the range-Doppler maps (RDMs) over different frequency bands, which severely limits the processing gain of MF integration. In this paper, a novel MTD algorithm is proposed to achieve both long-time integration and quasi-coherent MF integration. More specifically, the proposed method consists of two main steps, where a modified Radon–Fourier transform (RFT), termed as MF-based RFT (MF-RFT), is, firstly, used to eliminate the Doppler spread via designing a sequential of MF-based Doppler filter banks. Following the MF-RFT, a phase-compensation-based method is also developed to further remove the residual phase errors. This method involves formulating an optimization problem based on the minimum-entropy criterion and employing a particle swarm optimization (PSO) algorithm to solve it, after which quasi-coherent MF integration can be achieved with robustness. Both numerical results and field test results based on digital video broadcasting-satellite (DVB-S) signals demonstrate that the proposed algorithm outperforms the existing methods in the scenario of weak MTD.
Full article
(This article belongs to the Special Issue Breakthroughs in Passive Radar Technologies)
Open AccessArticle
Novel UAV Flight Designs for Accuracy Optimization of Structure from Motion Data Products
by
, , , , , , , and
Remote Sens. 2023, 15(17), 4308; https://doi.org/10.3390/rs15174308 (registering DOI) - 31 Aug 2023
Abstract
Leveraging low-cost drone technology, specifically the DJI Mini 2, this study presents an innovative method for creating accurate, high-resolution digital surface models (DSMs) to enhance topographic mapping with off-the-shelf components. Our research, conducted near Jena, Germany, introduces two novel flight designs, the “spiral”
[...] Read more.
Leveraging low-cost drone technology, specifically the DJI Mini 2, this study presents an innovative method for creating accurate, high-resolution digital surface models (DSMs) to enhance topographic mapping with off-the-shelf components. Our research, conducted near Jena, Germany, introduces two novel flight designs, the “spiral” and “loop” flight designs, devised to mitigate common challenges in structure from motion workflows, such as systematic doming and bowling effects. The analysis, based on height difference products with a lidar-based reference, and curvature estimates, revealed that “loop” and “spiral” flight patterns were successful in substantially reducing these systematic errors. It was observed that the novel flight designs resulted in DSMs with lower curvature values compared to the simple nadir or oblique flight patterns, indicating a significant reduction in distortions. The results imply that the adoption of novel flight designs can lead to substantial improvements in DSM quality, while facilitating shorter flight times and lower computational needs. This work underscores the potential of consumer-grade unoccupied aerial vehicle hardware for scientific applications, especially in remote sensing tasks.
Full article
(This article belongs to the Section Remote Sensing Image Processing)
►▼
Show Figures
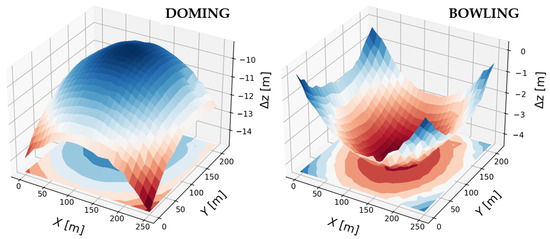
Figure 1
Open AccessArticle
Analysis of Mesoscale Eddy Merging in the Subtropical Northwest Pacific Using Satellite Remote Sensing Data
Remote Sens. 2023, 15(17), 4307; https://doi.org/10.3390/rs15174307 (registering DOI) - 31 Aug 2023
Abstract
►▼
Show Figures
Mesoscale eddies are ubiquitous in the ocean, yet our understanding of their evolutions, particularly eddy merging processes, remains enigmatic. In this study, the merging processes of two cyclonic–cyclonic and two anticyclonic–anticyclonic eddies are analyzed in the Subtropical Northwest Pacific using satellite remote sensing
[...] Read more.
Mesoscale eddies are ubiquitous in the ocean, yet our understanding of their evolutions, particularly eddy merging processes, remains enigmatic. In this study, the merging processes of two cyclonic–cyclonic and two anticyclonic–anticyclonic eddies are analyzed in the Subtropical Northwest Pacific using satellite remote sensing altimetry data. The results reveal that, as eddies approach each other, their contours become connected, leading to the formation of multi-core eddies. Simultaneously, the merging process prompts substantial exchanges of energy and vorticity, resulting in the dissipation of one eddy and the emergence of a more extensive merged eddy. Throughout the merging process, the eddy contours elongate upwards along the centerline (the line connecting eddy centers) and there are distinct changes in both the horizontal and vertical morphology of the eddies. Notably, after the merging, the eddies distinctly exhibit intensified signals of sea surface temperature and vertical temperature anomaly, an outcome of their transformative fusion. The findings of this study significantly enhance our understanding of mesoscale eddy dynamics, particularly in the intricate eddy merging process. However, it is important to note that, due to limitations in vertical observational data, this study does not provide a quantitative portrayal of the vertical mechanisms of eddy merging, which also underscores a pivotal avenue for future research in the field.
Full article
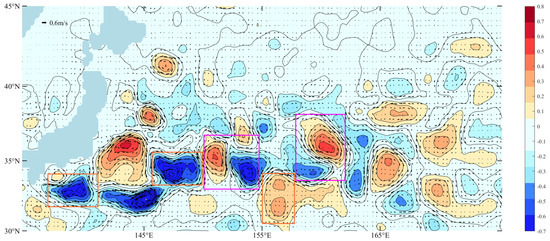
Figure 1
Open AccessArticle
A Flexible Spatiotemporal Thick Cloud Removal Method with Low Requirements for Reference Images
Remote Sens. 2023, 15(17), 4306; https://doi.org/10.3390/rs15174306 (registering DOI) - 31 Aug 2023
Abstract
Thick cloud and shadows have a significant impact on the availability of optical remote sensing data. Although various methods have been proposed to address this issue, they still have some limitations. First, most approaches rely on a single clear reference image as complementary
[...] Read more.
Thick cloud and shadows have a significant impact on the availability of optical remote sensing data. Although various methods have been proposed to address this issue, they still have some limitations. First, most approaches rely on a single clear reference image as complementary information, which becomes challenging when the target image has large missing areas. Secondly, the existing methods that can utilize multiple reference images require the complementary data to have high temporal correlation, which is not suitable for situations where the difference between the reference image and the target image is large. To overcome these limitations, a flexible spatiotemporal deep learning framework based on generative adversarial networks is proposed for thick cloud removal, which allows for the use of three arbitrary temporal images as references. The framework incorporates a three-step encoder that can leverage the uncontaminated information from the target image to assimilate the reference images, enhancing the model’s ability to handle reference images with diverse temporal differences. A series of simulated and real experiments on Landsat 8 and Sentinel 2 data is performed to demonstrate the effectiveness of the proposed method. The proposed method is especially applicable to small/large-scale regions with reference images that are significantly different from the target image.
Full article
(This article belongs to the Special Issue Advanced Learning Techniques for Remote Sensing Image Quality Improvement)
Open AccessArticle
Enhancing UAV-SfM Photogrammetry for Terrain Modeling from the Perspective of Spatial Structure of Errors
by
, , , , , and
Remote Sens. 2023, 15(17), 4305; https://doi.org/10.3390/rs15174305 (registering DOI) - 31 Aug 2023
Abstract
UAV-SfM photogrammetry is widely used in remote sensing and geoscience communities. Scholars have tried to optimize UAV-SfM for terrain modeling based on analysis of error statistics like root mean squared error (RMSE), mean error (ME), and standard deviation (STD). However, the errors of
[...] Read more.
UAV-SfM photogrammetry is widely used in remote sensing and geoscience communities. Scholars have tried to optimize UAV-SfM for terrain modeling based on analysis of error statistics like root mean squared error (RMSE), mean error (ME), and standard deviation (STD). However, the errors of terrain modeling tend to be spatially distributed. Although the error statistic can represent the magnitude of errors, revealing spatial structures of errors is still challenging. The “best practice” of UAV-SfM is lacking in research communities from the perspective of spatial structure of errors. Thus, this study designed various UAV-SfM photogrammetric scenarios and investigated the effects of image collection strategies and GCPs on terrain modeling. The error maps of different photogrammetric scenarios were calculated and quantitatively analyzed by ME, STD, and Moran’s I. The results show that: (1) A high camera inclination (20–40°) enhances UAV-SfM photogrammetry. This not only decreases the magnitude of errors, but also mitigates its spatial correlation (Moran’s I). Supplementing convergent images is valuable for reducing errors in a nadir camera block, but it is unnecessary when the image block is with a high camera angle. (2) Flying height increases the magnitude of errors (ME and STD) but does not affect the spatial structure (Moran’s I). By contrast, the camera angle is more important than the flying height for improving the spatial structure of errors. (3) A small number of GCPs rapidly reduce the magnitude of errors (ME and STD), and a further increase in GCPs has a marginal effect. However, the structure of errors (Moran’s I) can be further improved with increasing GCPs. (4) With the same number, the distribution of GCPs is critical for UAV-SfM photogrammetry. The edge distribution should be first considered, followed by the even distribution. The research findings contribute to understanding how different image collection scenarios and GCPs can influence subsequent terrain modeling accuracy, precision, and spatial structure of errors. The latter (spatial structure of errors) should be routinely assessed in evaluations of the quality of UAV-SfM photogrammetry.
Full article
(This article belongs to the Topic Advances, Innovations and Applications of UAV Technology for Remote Sensing)
►▼
Show Figures
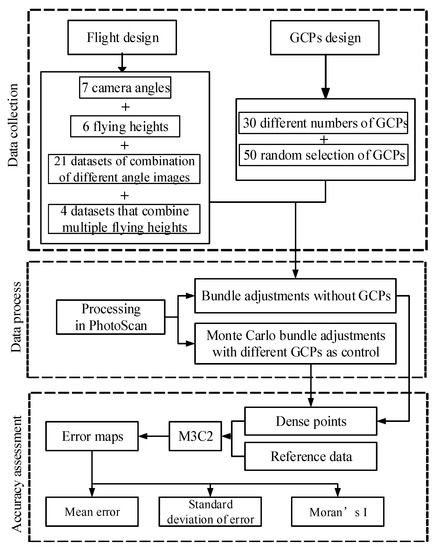
Figure 1
Open AccessArticle
Insight into the Characteristics and Triggers of Loess Landslides during the 2013 Heavy Rainfall Event in the Tianshui Area, China
Remote Sens. 2023, 15(17), 4304; https://doi.org/10.3390/rs15174304 - 31 Aug 2023
Abstract
The 2013 heavy rainfall event (from June to July) in the Tianshui area triggered the most serious rainfall-induced group-occurring landslides since 1984, causing extensive casualties and economic losses. To better understand the characteristics and triggers of these loess landslides, we conducted a detailed
[...] Read more.
The 2013 heavy rainfall event (from June to July) in the Tianshui area triggered the most serious rainfall-induced group-occurring landslides since 1984, causing extensive casualties and economic losses. To better understand the characteristics and triggers of these loess landslides, we conducted a detailed analysis of the landslides and relevant influencing factors. Based on the detailed rainfall-induced landslide database obtained using visual interpretation of remote sensing images before and after rainfall, the correlation between the landslide occurrence and different influencing factors such as terrain, geomorphology, geology, and rainfall condition was analyzed. This rainfall event triggered approximately 54,000 landslides with a total area of 67.9 km2, mainly consisting of shallow loess landslides with elongated type, shallow rockslides, collapses, and mudflows. The landslides exhibited a clustered distribution, with the majority concentrated in two specific areas (i.e., Niangniangba and Shetang). The abundance index of landslides was closely associated with the hillslope gradient, total rainfall, and drainage (river) density. The landslide area density (LAD) was positively correlated with these influential factors, characterized by either an exponential or a linear relationship. The Middle Devonian Shujiaba formation (D2S) was identified to be highly susceptible to landslides, and the landslide events therein accounted for 35% of the total landslide occurrences within 22% of the study area. In addition, the E-SE aspect was more prone to landslides, while the W-NW aspect exhibited a low abundance of landslides.
Full article
(This article belongs to the Special Issue Anticipation of Flash Floods and Rainfall-Induced Hydro-Geomorphic Hazards)
►▼
Show Figures
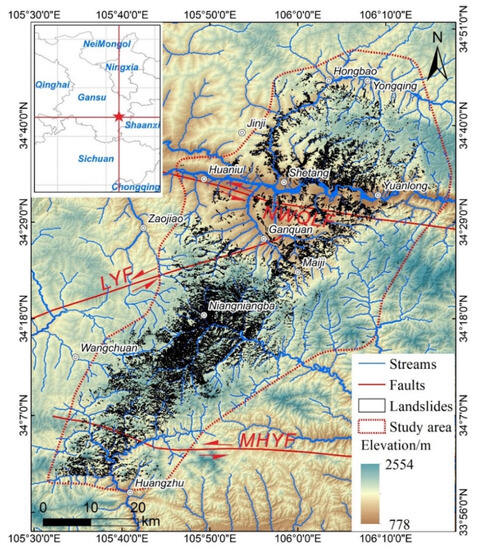
Figure 1
Open AccessArticle
Carotenoid Content Estimation in Tea Leaves Using Noisy Reflectance Data
by
and
Remote Sens. 2023, 15(17), 4303; https://doi.org/10.3390/rs15174303 - 31 Aug 2023
Abstract
Quantifying carotenoid content in agriculture is essential for assessing crop nutritional value, improving crop quality, promoting human health, understanding plant stress responses, and facilitating breeding and genetic improvement efforts. Hyperspectral reflectance imaging is a nondestructive and rapid tool for estimating the carotenoid content.
[...] Read more.
Quantifying carotenoid content in agriculture is essential for assessing crop nutritional value, improving crop quality, promoting human health, understanding plant stress responses, and facilitating breeding and genetic improvement efforts. Hyperspectral reflectance imaging is a nondestructive and rapid tool for estimating the carotenoid content. In spectrometer reflectance measurements, there are various sources of noise that can compromise the accuracy of carotenoid content estimations. Recently, various machine learning algorithms have been identified as robust against various types of noise, eliminating the need for denoising processes. Specifically, Cubist and the one-dimensional convolutional neural network (1D-CNN) have been used in evaluating vegetation properties based on reflectance data. We used regression models based on Cubist and 1D-CNN to estimate carotenoid content from reflectance data (the spectral resolution was resampled in 5 nm bands across the entire wavelength domain from 400 to 850 nm) with various degrees of Gaussian and spike noise added. The Cubist-based model was the most robust for this purpose: it achieved a ratio of performance to deviation of 1.41, a root mean square error of 1.11 µg/cm2, and a coefficient of determination (R2) of 0.496 when applied to reflectance data with a combination of Gaussian (mean: 0; variance: 0.04) and spike noise (density: 0.05; amplitude: 0.05).
Full article
(This article belongs to the Special Issue Advances in Remote Sensing of Vegetation Traits Retrieval Based on Hyperspectral Data Analysis)
►▼
Show Figures
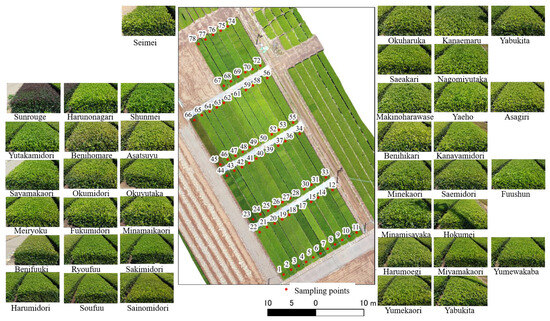
Figure 1
Open AccessArticle
Comparative Analysis of Gully Morphology Extraction Suitability Using Unmanned Aerial Vehicle and Google Earth Imagery
Remote Sens. 2023, 15(17), 4302; https://doi.org/10.3390/rs15174302 - 31 Aug 2023
Abstract
Gully erosion is considered to be a highly destructive form of soil erosion, often leading to the occurrence of natural calamities like landslides and mudslides. Remote sensing images have been extensively utilized in gully erosion research, and the suitability of extracting gully morphology
[...] Read more.
Gully erosion is considered to be a highly destructive form of soil erosion, often leading to the occurrence of natural calamities like landslides and mudslides. Remote sensing images have been extensively utilized in gully erosion research, and the suitability of extracting gully morphology parameters in various topographic regions needs to be clarified. Based on field measurements, this paper focuses on two widely used high-resolution remote sensing images: Unmanned Aerial Vehicle (UAV) and Google Earth (GE) imagery. It systematically examines the accuracy of gully morphological characteristic extraction using remote sensing in two regions with different terrain characteristics. The results show the following: (1) Compared to interpreting wide gullies with unclear shoulder lines, centimeter-level UAV imagery is more suitable for interpreting narrow gullies with clear shoulder lines. Conversely, the interpretability of sub-meter-level GE imagery is exactly the opposite. (2) The error in interpreting gully head points (GHPs) based on UAV images is less than 1 m, while the errors in gully length (GL), width (GW), perimeter (GP) and area (GA) are all below 3%, and these errors are hardly affected by gully morphology. (3) The error of GHPs based on GE images is concentrated within the range of 1–3 m. Meanwhile, the errors associated with GL, GP and GA are less than 10%. Conversely, the error of GW exceeds 11%. Furthermore, the aforementioned errors tend to increase as the gully width decreases and the complexity of the gully shoulder line increases. These findings shed light on the suitability of two commonly used remote sensing images for gully morphology extraction and provide valuable guidance for image selection in future research endeavors in this field.
Full article
(This article belongs to the Special Issue Mapping and Monitoring of Geohazards with Remote Sensing Technologies II)
►▼
Show Figures
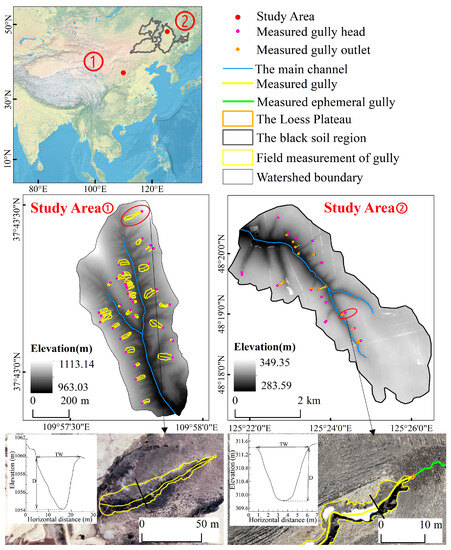
Figure 1
Open AccessArticle
Spatiotemporal Information Mining for Emergency Response of Urban Flood Based on Social Media and Remote Sensing Data
Remote Sens. 2023, 15(17), 4301; https://doi.org/10.3390/rs15174301 - 31 Aug 2023
Abstract
The emergency response is crucial in preventing and mitigating urban floods. Both remote sensing and social media data offer distinct advantages in large-scale flood monitoring and near-real-time flood monitoring. However, current research lacks a thorough exploration of the application of social media data
[...] Read more.
The emergency response is crucial in preventing and mitigating urban floods. Both remote sensing and social media data offer distinct advantages in large-scale flood monitoring and near-real-time flood monitoring. However, current research lacks a thorough exploration of the application of social media data and remote sensing imagery in the urban flood emergency response. To address this issue, this paper, while extracting disaster information based on social media data, deeply mines the spatiotemporal distribution characteristics and dynamic spatial accessibility of rescue points. Furthermore, SAR imagery and social media data for monitoring urban flooding are compared. This study took the Zhengzhou 7.20 urban flood as a case study and created a methodological framework to quickly extract flood disaster information (flood, landslide, and rescue points) using these two types of data; spatiotemporal analysis and random forest classification were also conducted to mine valuable information. Temporally, the study revealed that disaster information did not increase proportionally with the amount of rainfall during the rainfall process. Spatially, specific regions with higher susceptibility to flooding, landslides, and rescue points were identified, such as the central region characterized by low drainage standards and high-density urban areas, as well as the eastern region with low-lying terrain. Moreover, this study examined the spatial accessibility of rescue resources in real flood scenarios and found that their service coverage varied throughout the day during and after the disaster. In addition, social media excelled in high-density urban areas’ flood point extraction, while SAR performed better in monitoring floods at the edges of low-density urban areas and large water bodies, allowing them to complement each other, to a certain extent. The findings of this study provide scientific reference value for the optimal selection of rescue paths and the allocation of resources in the emergency response to urban floods caused by extreme rainstorms.
Full article
(This article belongs to the Special Issue Remote Sensing Applications for Flood Forecasting and Flood Risk Management)
►▼
Show Figures
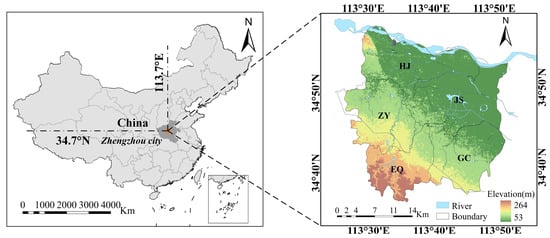
Figure 1
Open AccessArticle
A Multi-Correlation Peak Phase Deblurring Algorithm for BeiDou B1C Signals in Urban Environments
Remote Sens. 2023, 15(17), 4300; https://doi.org/10.3390/rs15174300 - 31 Aug 2023
Abstract
With the widespread global application of BeiDou navigation, BeiDou B1C signaling based on Quadrature Multiplexed Binary Offset Carrier (QMBOC) modulation is expected to be extensively used in urban environments due to its wider signal bandwidth, smaller code pseudorange measurement errors, and stronger multipath
[...] Read more.
With the widespread global application of BeiDou navigation, BeiDou B1C signaling based on Quadrature Multiplexed Binary Offset Carrier (QMBOC) modulation is expected to be extensively used in urban environments due to its wider signal bandwidth, smaller code pseudorange measurement errors, and stronger multipath capabilities. Despite offering higher positioning accuracy and secondary modulation characteristics of the BeiDou, B1C signals introduce the challenge of multiple peaks in the autocorrelation function. This leads to phase ambiguity during signal acquisition and tracking, resulting in positioning deviations of tens or even hundreds of meters. In urban environments, such deviations give rise to significant practical application issues. To address this problem, we have designed a multi-loop structure for the synchronous tracking of B1C signals and proposed a multi-peak phase-deblurring algorithm specifically tailored for the BeiDou B1C signal in urban environments. This algorithm considers the coupling relationship between the code and the carrier loops, and by matching the structural design of multiple loops, it achieves a precise and unambiguous phase estimation of the pseudocode, enabling the stable tracking of the entire loop for the BeiDou B1C signal. Simulation and actual testing demonstrate that the algorithm exhibits an error less than 0.03 for chip intervals when the signal-to-noise ratio is greater than −20 dB. Additionally, the accuracy can be improved by adjusting the set conditions, making it suitable for urban environments.
Full article
(This article belongs to the Special Issue GNSS for Urban Transport Applications II)
►▼
Show Figures
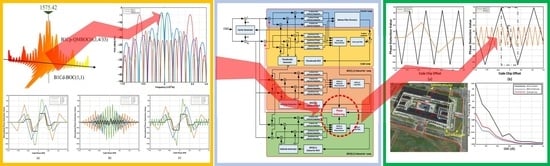
Graphical abstract
Open AccessArticle
Spectral De-Aliasing Method of Micro-Motion Signals Based on a Complex-Valued U-Net Network
Remote Sens. 2023, 15(17), 4299; https://doi.org/10.3390/rs15174299 - 31 Aug 2023
Abstract
Spectrum aliasing occurs in signal echoes when the sampling frequency does not comply with the Nyquist Sampling Theorem. In this scenario, the extraction of micro-motion parameters becomes challenging. This paper proposes a spectral de-aliasing method for micro-motion signals based on a complex-valued U-Net
[...] Read more.
Spectrum aliasing occurs in signal echoes when the sampling frequency does not comply with the Nyquist Sampling Theorem. In this scenario, the extraction of micro-motion parameters becomes challenging. This paper proposes a spectral de-aliasing method for micro-motion signals based on a complex-valued U-Net network. Zero interpolation is employed to insert zeros into the echo, effectively increasing the sampling frequency. After zero interpolation, the micro-motion signal contains both real micro-motion signal frequency components and new frequency components. Short-Time Fourier Transform (STFT) is then applied to transform the zero-interpolated echo from the time domain to the time–frequency domain. Furthermore, a complex-valued U-Net training model is utilized to eliminate redundant frequency components generated by zero interpolation, thereby achieving the frequency reconstruction of micro-motion signal echoes. Finally, the training models are employed to process the measured data. The theoretical analysis, simulations, and experimental results demonstrate that this method is robust and feasible, and is capable of addressing the problem of micro-motion signal echo spectrum aliasing in narrowband radar.
Full article
(This article belongs to the Special Issue Doppler Radar: Signal, Data and Applications)
►▼
Show Figures
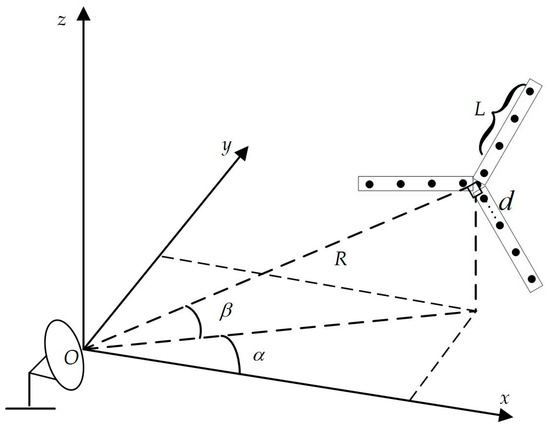
Figure 1
Open AccessArticle
Remotely Sensed Agriculture Drought Indices for Assessing the Impact on Cereal Yield
by
, , , and
Remote Sens. 2023, 15(17), 4298; https://doi.org/10.3390/rs15174298 - 31 Aug 2023
Abstract
►▼
Show Figures
This study aims to analyze the potential of different drought indices for identifying drought periods and predicting cereal yield in two semi-arid regions, Lleida in Catalonia and Kairouan in Tunisia, which have similar Mediterranean climates but different agricultural practices. Four drought indices, namely
[...] Read more.
This study aims to analyze the potential of different drought indices for identifying drought periods and predicting cereal yield in two semi-arid regions, Lleida in Catalonia and Kairouan in Tunisia, which have similar Mediterranean climates but different agricultural practices. Four drought indices, namely the Soil Moisture Anomaly Index (SMAI), the Vegetation Anomaly Index (VAI), the Evapotranspiration Anomaly Index (EAI), and the Inverse Temperature Anomaly Index (ITAI), were calculated from remote sensing data. Drought periods were identified from 2010/2011 to 2021/2022 based on the aforementioned indices. A correlation study between drought indices and wheat and barley yields was performed in order to select the most informative index and month for yield prediction. In the rainfed cereal area of Lleida, the strongest correlation was found between the EAI and VAI with barley yield (0.91 and 0.83, respectively) at the time of cereal maturity in June. For wheat, the strongest correlation was found between the EAI and VAI (0.75 and 0.72, respectively) at the time of cereal maturity in July. However, the VAI, EAI, and SMAI showed the best performance as an earlier indicator in March with a correlation with barley yield of 0.72, 0.67, and 0.64, respectively; the lowest standard deviation was for the SMAI. For wheat yield, the best earlier indicator was the SMAI in March, showing the highest correlation (0.6) and the lowest standard deviation. For the irrigated cereal zone of Kairouan, the strongest correlation (0.9) and the lowest standard deviation are found between the EAI and cereal yield in April. In terms of advanced prediction, the VAI shows a high correlation in March (0.79) while the SMAI shows a slightly lower correlation in February (0.67) and a lower standard deviation. The results highlight the importance of the EAI and SMAI as key indicators for the estimation and early estimation (respectively) of cereal yield.
Full article
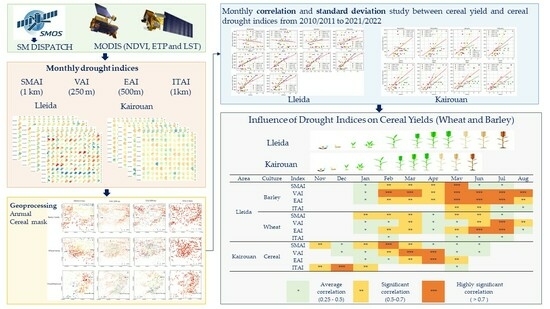
Graphical abstract
Open AccessArticle
Sat-Mesh: Learning Neural Implicit Surfaces for Multi-View Satellite Reconstruction
by
and
Remote Sens. 2023, 15(17), 4297; https://doi.org/10.3390/rs15174297 - 31 Aug 2023
Abstract
Automatic reconstruction of surfaces from satellite imagery is a hot topic in computer vision and photogrammetry. State-of-the-art reconstruction methods typically produce 2.5D elevation data. In contrast, we propose a one-stage method directly generating a 3D mesh model from multi-view satellite imagery. We introduce
[...] Read more.
Automatic reconstruction of surfaces from satellite imagery is a hot topic in computer vision and photogrammetry. State-of-the-art reconstruction methods typically produce 2.5D elevation data. In contrast, we propose a one-stage method directly generating a 3D mesh model from multi-view satellite imagery. We introduce a novel Sat-Mesh approach for satellite implicit surface reconstruction: We represent the scene as a continuous signed distance function (SDF) and leverage a volume rendering framework to learn the SDF values. To address the challenges posed by lighting variations and inconsistent appearances in satellite imagery, we incorporate a latent vector in the network architecture to encode image appearances. Furthermore, we introduce a multi-view stereo constraint to enhance surface quality. This constraint minimizes the similarity between image patches to optimize the position and orientation of the SDF surface. Experimental results demonstrate that our method achieves superior visual quality and quantitative accuracy in generating mesh models. Moreover, our approach can learn seasonal variations in satellite imagery, resulting in texture mesh models with different and consistent seasonal appearances.
Full article
(This article belongs to the Special Issue 3D Information Recovery and 2D Image Processing for Remotely Sensed Optical Images)
►▼
Show Figures
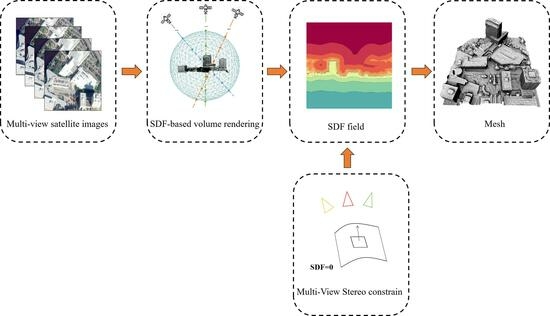
Graphical abstract
Open AccessArticle
A Random Forest Algorithm Combined with Bayesian Optimization for Atmospheric Duct Estimation
Remote Sens. 2023, 15(17), 4296; https://doi.org/10.3390/rs15174296 - 31 Aug 2023
Abstract
►▼
Show Figures
Inversion of atmospheric ducts is of great importance in the field of performance evaluation for radar and communication systems. Since the model parameters in machine learning play a crucial role in prediction performance, this paper develops a random forest (RF) model integrated with
[...] Read more.
Inversion of atmospheric ducts is of great importance in the field of performance evaluation for radar and communication systems. Since the model parameters in machine learning play a crucial role in prediction performance, this paper develops a random forest (RF) model integrated with Bayesian optimization (BO) called BO-RF for atmospheric duct prediction, and the BO is adopted to determine appropriate model parameters during the training process. In addition, the K-fold cross-validation (CV) method is also incorporated into the model to obtain the best model partition and overcome the overfitting problem. To test the performance of the proposed model, the results obtained by the BO-RF are compared with other commonly used methods, such as classical RF, extreme gradient boosting (XGBoost) with/without BO, and K-nearest neighbor (KNN) with/without BO. Comparisons demonstrate that BO-RF has the best accuracy and anti-noise ability for the estimation of duct parameters.
Full article
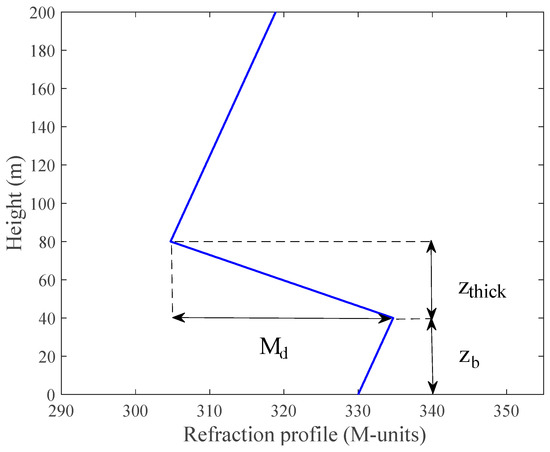
Figure 1
Open AccessArticle
Detection Method of Infected Wood on Digital Orthophoto Map–Digital Surface Model Fusion Network
by
, , , , , and
Remote Sens. 2023, 15(17), 4295; https://doi.org/10.3390/rs15174295 - 31 Aug 2023
Abstract
Pine wilt disease (PWD) is a worldwide affliction that poses a significant menace to forest ecosystems. The swift and precise identification of pine trees under infection holds paramount significance in the proficient administration of this ailment. The progression of remote sensing and deep
[...] Read more.
Pine wilt disease (PWD) is a worldwide affliction that poses a significant menace to forest ecosystems. The swift and precise identification of pine trees under infection holds paramount significance in the proficient administration of this ailment. The progression of remote sensing and deep learning methodologies has propelled the utilization of target detection and recognition techniques reliant on remote sensing imagery, emerging as the prevailing strategy for pinpointing affected trees. Although the existing object detection algorithms have achieved remarkable success, virtually all methods solely rely on a Digital Orthophoto Map (DOM), which is not suitable for diseased trees detection, leading to a large false detection rate in the detection of easily confused targets, such as bare land, houses, brown herbs and so on. In order to improve the ability of detecting diseased trees and preventing the spread of the epidemic, we construct a large-scale PWD detection dataset with both DOM and Digital Surface Model (DSM) images and propose a novel detection framework, DDNet, which makes full use of the spectral features and geomorphological spatial features of remote sensing targets. The experimental results show that the proposed joint network achieves an AP50 2.4% higher than the traditional deep learning network.
Full article
(This article belongs to the Special Issue Advanced Artificial Intelligence for Remote Sensing: Methodology and Applications)
►▼
Show Figures
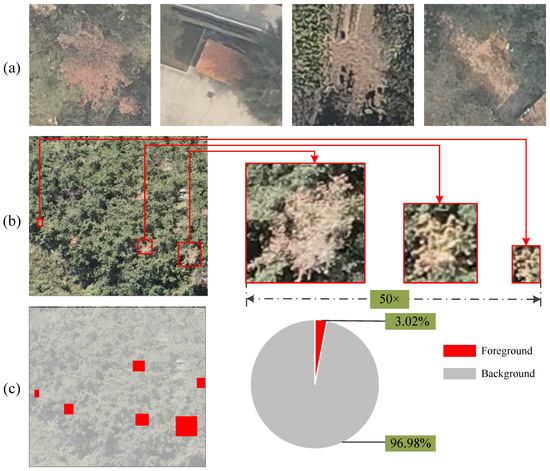
Figure 1
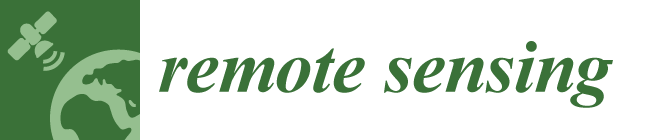
Journal Menu
► ▼ Journal Menu-
- Remote Sensing Home
- Aims & Scope
- Editorial Board
- Reviewer Board
- Topical Advisory Panel
- Photography Exhibition
- Instructions for Authors
- Special Issues
- Topics
- Sections & Collections
- Article Processing Charge
- Indexing & Archiving
- Editor’s Choice Articles
- Most Cited & Viewed
- Journal Statistics
- Journal History
- Journal Awards
- Society Collaborations
- Conferences
- Editorial Office
Journal Browser
► ▼ Journal BrowserHighly Accessed Articles
Latest Books
E-Mail Alert
News
Topics
Topic in
Machines, Micromachines, Remote Sensing, Sustainability, Symmetry, Wind
Energy Equipment and Condition Monitoring
Topic Editors: Zhifeng Xiao, Bin Huang, Lida LiaoDeadline: 15 September 2023
Topic in
AI, Applied Sciences, BDCC, Remote Sensing, Sensors
Deep Learning and Transformers’ Methods Applied to Remotely Captured Data
Topic Editors: Moulay A. Akhloufi, Mozhdeh ShahbaziDeadline: 30 September 2023
Topic in
Geosciences, Hydrology, IJERPH, Remote Sensing, Water
Groundwater Pollution Control and Groundwater Management
Topic Editors: Yunhui Zhang, Qili Hu, Liting HaoDeadline: 31 October 2023
Topic in
Energies, Hydrology, Remote Sensing, Sustainability, Water
Human Impact on Groundwater Environment
Topic Editors: Zongjun Gao, Jiutan LiuDeadline: 15 November 2023

Conferences
Special Issues
Special Issue in
Remote Sensing
Remote Sensing in Coastal Ecosystem Monitoring
Guest Editors: Valeria Tomaselli, Maria Adamo, Cristina TarantinoDeadline: 1 September 2023
Special Issue in
Remote Sensing
Dam Stability Monitoring with Satellite Geodesy
Guest Editors: Antonio Miguel Ruiz Armenteros, Roberto Tomás, Joaquim João Sousa, M. Clara de Lacy, Zhenhong LiDeadline: 15 September 2023
Special Issue in
Remote Sensing
Recent Advances of Remote Sensing in Monitoring Agro-Meteorological Disasters
Guest Editors: Koki Homma, Masayasu Maki, Mongkol RaksapatcharawongDeadline: 30 September 2023
Special Issue in
Remote Sensing
Hyperspectral Remote Sensing from Spaceborne and Low Altitude Aerial/Drone-Based Platforms — Differences in Approaches, Data Processing Methods, and Applications
Guest Editors: Amin Beiranvand Pour, Arindam Guha, Laura Crispini, Snehamoy ChatterjeeDeadline: 15 October 2023
Topical Collections
Topical Collection in
Remote Sensing
Google Earth Engine Applications
Collection Editors: Lalit Kumar, Onisimo Mutanga
Topical Collection in
Remote Sensing
Feature Papers for Section Environmental Remote Sensing
Collection Editor: Magaly Koch
Topical Collection in
Remote Sensing
Discovering A More Diverse Remote Sensing Discipline
Collection Editors: Gopika Suresh, Kate C. Fickas, Karen Joyce, Meghan Halabisky, Cristina Gómez, Michelle Kalamandeen
Topical Collection in
Remote Sensing
Current, Planned, and Future Satellite Missions: Guidelines for Data Exploitation by the Remote Sensing Community
Collection Editors: Jose Moreno, Magaly Koch, Robert Wang